I want you to imagine what life was like before we had object storage. Uploading
files was a custom process. If you wanted to scale, you ended up having to hire
storage area network experts that built complicated systems with terms like
“LUN” and “erasure coding”. Your application had to either shell out to an FTP
server to handle uploads or put them alongside the source code. Above all
though: everything had to be planned in advance. You had to do capacity planning
so that you could know how much storage you needed to buy and when you needed to
buy it. You couldn’t just insert a credit card and then get all the storage you
wanted.
In 2006, Amazon invented the concept of object storage, fundamentally changing
how applications work. Storage became a faucet. If you want more, you simply
turn the knob. Bottomless storage was revolutionary, and now we’ve come to
expect storage to be decoupled from physical hardware. S3 paved the way for
seamless data management across any environment as long as it had a connection
back to Amazon.
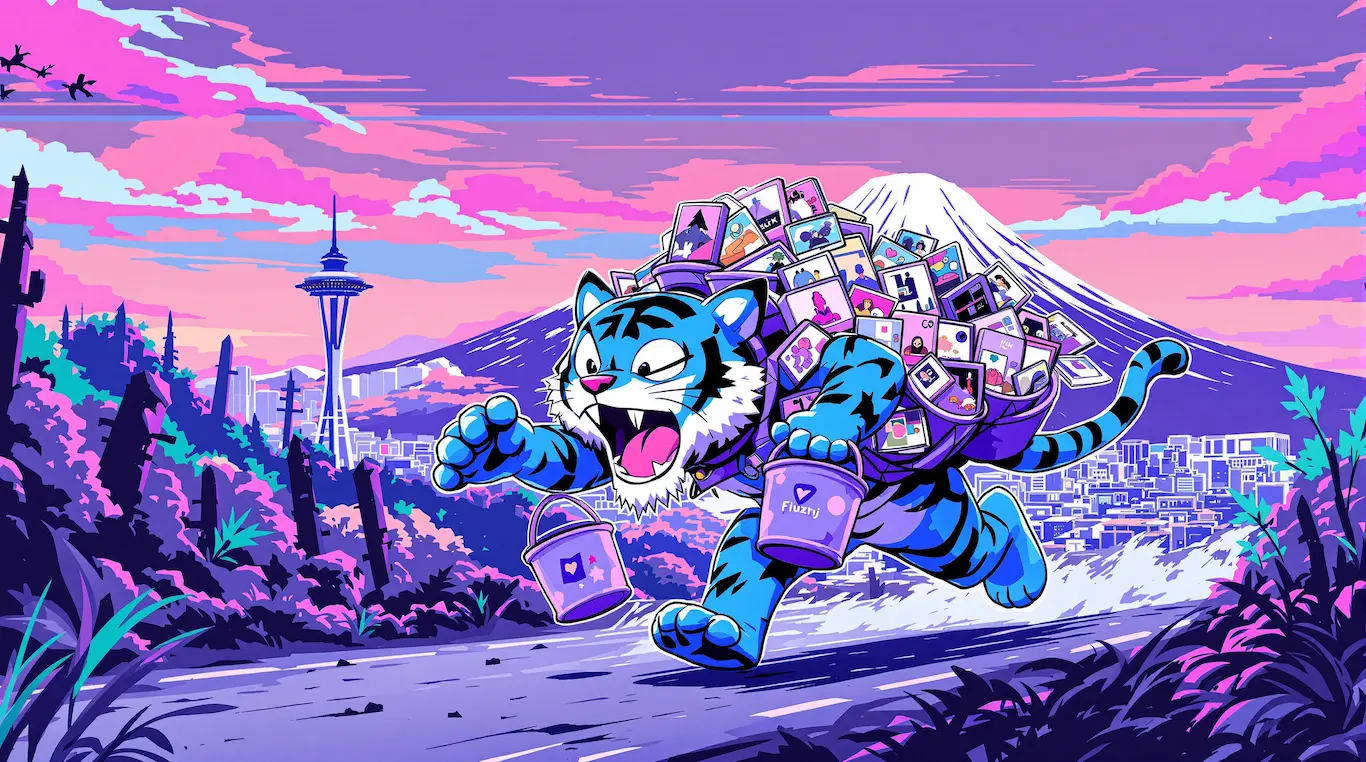
An anthropomorphic tiger running between datacentres. Image generated
using Flux pro [ultra] on fal.ai.